Adaptability and stability of soybean [Glycine max (L.) Merrill] strains in Central-West Brazil
DOI:
https://doi.org/10.14295/bjs.v3i7.594Keywords:
productivity, genotypes, environments, selectionAbstract
Soybean (Glycine max (L.) Merril) is one of the most important seed legumes in the world due to its high protein and vegetable oil content, being widely used in the food industry and animal feed. However, environmental changes, especially those related to rising global temperatures due to CO2 emissions, are impacting plant productivity, including soybeans. In this context, genetic improvement programs have been fundamental to develop cultivars that are more resistant to abiotic stresses, such as droughts and intense rains. To evaluate the adaptability and stability of soybean genotypes in different environments, statistical methods such as AMMI (Multiplicative Model of Interpretation and Intersection) and BLUP (Best Linear Unbased Prediction) have been widely used. The AMMI model is used to analyze the interaction between genotype and environment, while the BULP considers random genetic effects, providing a more accurate estimate of genetic value. Furthermore, the weighting between stability (WAASBY) (Weighted Average WAASB) index has been used to identify stable, high-performance genotypes, combining stability and yield characteristics. In the research carried out, eleven improved soybean lines were evaluated in five municipalities in the central-western region of Brazil. Data were analyzed using statistical techniques such as linear mixed model, GGE biplot and AMMI and BLUP models. The results indicated that soybean lines showed significant variations in productivity in different environments, highlighting specific genotypes for each location. The productivity prediction analysis showed that the BLUP model was more accurate compared to the AMMI model. Furthermore, the GGE biplot identified the most suitable genotypes for each environment, considering both average performance and stability. Finally, the combination of characteristics from the AMMI and BLUP techniques, using the WAASBY index, made it possible to identify genotypes with high potential for yield and stability. In summary, the results of this research contribute to the development of soybean cultivars more adapted to variable environmental conditions, providing valuable information for genetic improvement programs and agricultural practices in the central-western region of Brazil. The integration of different statistical methods and evaluation indices has been fundamental to improving the selection of genotypes with high performance and stability, contributing to food security and the sustainability of agricultural production.
References
Abdelghany, A. M., Zhang, S., Azam, M., Shaibu, A. S., Feng, Y., Qi, J., Li, J., Li, Y., Tian, Y., Hong, H., Lamlom, S. F., Li, B., & Sun, J. (2021). Exploring the phenotypic stability of soybean seed compositions using multi-trait stability index approach. Agronomy, 11(11), 2200. https://doi.org/10.3390/agronomy11112200. DOI: https://doi.org/10.3390/agronomy11112200
Ajay, V., Gyanendra, S. (2021). BLUP & AMMI model for stability analysis of wheat genotypes evaluated under irrigated timely sown trials in North Eastern Plains Zone of India. Journal of Plant Breeding and Crop Science, 13(3), 144-154. https://doi.org/10.5897/JPBCS2021.0947 DOI: https://doi.org/10.5897/JPBCS2021.0947
Al-Ballat, I. A., & Al-Araby, A. A. (2019). Correlation and path coefficient analysis for seed yield and some of its traits in common bean (Phaseolus vulgaris L.). Egyptian Journal of Horticulture, 46(1), 41-51. https://dx.doi.org/10.21608/ejoh.2018.6481.1088 DOI: https://doi.org/10.21608/ejoh.2018.6481.1088
Alencar, X., Jarquin, D., Howard, R., Ramasubramanian, V., Specht, J. E., Graed, G. L., Beavis, W. D., Diers, B. W., Song, Q., Cregan, P. B., Nelson, R., Mian, R., Shannon, J. G., McHalw, L., Wang, D., Schapaugh, W., Lorenz, A. J., Xu, S., Muir, W. M., & Rainey, K. M. (2018). Genome-wide analysis of grain yield stability and environmental interactions in a multiparental soybean population. G3 Genes, 8, 519-529. https://doi.org/10.1534/g3.117.300300. DOI: https://doi.org/10.1534/g3.117.300300
Alves, R. S., Rocha, J. R. A. S. C., Teodoro, P. E., Resende, M. D. V., Henriques, E. P., Silva, L. A., Carneiro, P. C. S., & Bhering, L. L. (2018). Multiple-trait BLUP: a suitable strategy for genetic selection of Eucalyptus. Tree Genetics & Genomes, 14, 77. https://doi.org/10.1007/s11295-018-1292-7 DOI: https://doi.org/10.1007/s11295-018-1292-7
Anisha, A., Rajappa, P. V., Parashuram, P., Hemalatha, V., Dhasyashree, R., Tonapi, V. A., Sujatha, K., Girish, G., & Madhusudhana, R. (2022). Selection of post-rainy sorghum landraces combining multi-traits mean performance and stability. Euphytica, 218(12), 176. DOI: https://doi.org/10.1007/s10681-022-03127-4
Anuradha, N., Patro, T. S. S. K., Singamsetti, A., Rani, Y. S., Triveni, U., Kumari, A. N., Govanakoppa, N., Pathy, T. T., & Tonapi, V. A. (2022). Comparative study of AMMI-and BLUP-based simultaneous selection for grain yield and stability of finger millet [Eleusine coracana (L.) Gaertn.] genotypes. Frontiers in plant science, 12, 786839. DOI: https://doi.org/10.3389/fpls.2021.786839
Ayed, S., Othmani, A., Bouhaouel, I., Teixeira da Silva, J. A. (2021). Multi-environment screening of durum wheat genotypes for drought tolerance in changing climatic events. Agronomy, 11(5), 875. https://doi.org/10.3390/agronomy11050875 DOI: https://doi.org/10.3390/agronomy11050875
Betrán, F. J., Bänziger, D. B. M., & Edmeades, G. O. (2003). Genetic analysis of inbred and hybrid grain yield under stress and nonstress environments in tropical maize. Crop Science, 43(3), 807-817. https://doi.org/10.2135/cropsci2003.8070 DOI: https://doi.org/10.2135/cropsci2003.8070
Conab - Companhia Nacional de Abastecimento. (2024). Acompanhamento da Safra Brasileira de Grãos, Brasília, DF, 11, safra 2023/24, n. 6 sexto levantamento.
Crossa, J., Cornelius, P. L., Yan, W. (2002). Biplots of linear‐bilinear models for studying crossover genotype× environment interaction. Crop Science, 42(2), 619-633. https://doi.org/10.2135/cropsci2002.6190 DOI: https://doi.org/10.2135/cropsci2002.6190
Daba, S. D., Kiszonas, A. M., & McGee, R. J. (2023). Selecting stable and high-performance pea genotypes in multi-environmental assay (MET): Applying AMMI, GGE-Biplot and BLUP procedures. Plants, 12(12), 2343. https://doi.org/10.3390/plants12122343. DOI: https://doi.org/10.3390/plants12122343
Enyew, M., Feyissa, T., Geleta, M., Tesfaye, K., Hammenhag, C., & Carlsson, A. S. (2021). Genotype by environment interaction, correlation, AMMI, GGE biplot and cluster analysis for grain yield and other agronomic traits in sorghum (Sorghum bicolor L. Moench). Plos One, 16(10), e0258211. https://doi.org/10.1371/journal.pone.0258211 DOI: https://doi.org/10.1371/journal.pone.0258211
Evangelista, J. S. P. C., Alves, R. S., Peixoto, M. A., Resende, M. D. V., Teodoro, P. E., Silva, F. L., & Bhering, L. L. (2021). Soybean productivity, stability, and adaptability through mixed model methodology. Ciência Rural, 51(2), e20200406. https://doi.org/10.1590/0103-8478cr20200406. DOI: https://doi.org/10.1590/0103-8478cr20200406
Gauch Jr, H. G. (1992). AMMI analysis on yield trials. CIMMYT Wheat Special Report (CIMMYT), 8.
Habtegebriel, M. H. (2022). Adaptability and stability for soybean yield by AMMI and GGE models in Ethiopia. Frontiers in Plant Science, 13, 950992. https://doi.org/10.3389/fpls.2022.950992 DOI: https://doi.org/10.3389/fpls.2022.950992
Happ, M. M. (2023). Method Developments to Identify Loci and Selection Patterns Associated with Genotype by Environment Interactions in Soybean. Doctorate Thesis in The University of Nebraska-Lincoln.
Hassani, M., Mahmoudi, S. B., Saremirad, A., & Taleghani, D. (2023). Genotype by environment and genotype by yield* trait interactions in sugar beet: analyzing yield stability and determining key traits association. Scientific Reports, 13(1), 23111. https://doi.org/10.1038/s41598-023-51061-9 DOI: https://doi.org/10.1038/s41598-023-51061-9
Hilmarsson, H. S., Rio, S., & Sánchez, J. I. (2021). Genotype by environment interaction analysis of agronomic spring barley traits in Iceland using AMMI, factorial regression model and linear mixed model. Agronomy, 11(3), 499. https://doi.org/10.3390/agronomy11030499 DOI: https://doi.org/10.3390/agronomy11030499
Hossain, A., Sarker, U., Azam, G., Kobir, S., Roychowdhury, R., Ercisli, S., Ali, D., Oba, S., & Golokhvast, K. S. (2023). Integrating BLUP, AMMI, and GGE models to explore GE interactions for adaptability and stability of winter lentils (Lens culinaris Medik.). Plants, 12(11), 2079. https://doi.org/10.3390/plants12112079 DOI: https://doi.org/10.3390/plants12112079
Hyten, D., Happ, M., Graef, G., & Howard, R. (2024). Variable selection patterns associated with constitutive and GxE effects for grain yield in a locally adapted soybean population. Research Square. https://doi.org/10.21203/rs.3.rs-4189809/v1 DOI: https://doi.org/10.21203/rs.3.rs-4189809/v1
Kebede, G., Worku, W., Feyissa, F., & Jifar, H. (2024). Grain yield stability analysis for oat (Avena sativa L.) genotypes using additive main effects and multiplicative interactions model under different environments in Ethiopia. Ecological Genetics and Genomics, 30, 100228. https://doi.org/10.1016/j.egg.2024.100228 DOI: https://doi.org/10.1016/j.egg.2024.100228
Kim, H. J., Ha, B-K., Ha, K-S., Chae, J-H., Park, J-H., Kim, M-S., Asekova, S., Shannon, J. G., Son, C-K., & Lee, J-D. (2015). Comparison of a high oleic acid soybean line to cultivated cultivars for seed yield, protein and oil concentrations. Euphytica, 201, 285-292. https://doi.org/10.1007/s10681-014-1210-5. DOI: https://doi.org/10.1007/s10681-014-1210-5
Koundinya A.V. V., Ajeesh, B. R., Hegde, V., Sheela, M. N., Mohan, C., & Asha, K. I. (2021). Genetic parameters, stability and selection of cassava genotypes between rainy and water stress conditions using AMMI, WAAS, BLUP and MTSI. Scientia Horticulturae, 281, 109949. https://doi.org/10.1016/j.scienta.2021.109949. DOI: https://doi.org/10.1016/j.scienta.2021.109949
Lee, S. Y., Lee, H-S., Lee, C-M., Ha, S-K., Park, H-M., Lee, S-M., Kwon, Y., Jesung, J-U., & Mo, Y. (2023). Multi-environment trials and stability analysis for yield-related traits of commercial rice cultivars. Agriculture, 13(2), 256. https://doi.org/10.3390/agriculture13020256 DOI: https://doi.org/10.3390/agriculture13020256
Li, M., Liu, Y., Wang, C., Yang, X., Li, D., Zhang, X., Xu, C., Zhang, Y., Li, W., & Zhao, L. (2020). Identification of traits contributing to high and stable yields in different soybean varieties across three Chinese latitudes. Frontiers in Plant Science, 10, 1642. https://doi.org/10.3389/fpls.2019.01642 DOI: https://doi.org/10.3389/fpls.2019.01642
Li, Z., & Wu, W. (2023). Genotype recommendations for high performance and stability based on multiple traits selection across a multi-environment in rapeseed. European Journal of Agronomy, 145, 126787. https://doi.org/10.1016/j.eja.2023.126787 DOI: https://doi.org/10.1016/j.eja.2023.126787
Malosetti, M., Ribaut, J-M., & Van Eeuwijk, F. A. (2013). The statistical analysis of multi-environment data: modeling genotype-by-environment interaction and its genetic basis. Frontiers in Physiology, 4, 37433. https://doi.org/10.3389/fphys.2013.00044 DOI: https://doi.org/10.3389/fphys.2013.00044
ManWah, L., DaWei, X., YiShu, G., KwanPok, L., KenJing, F., Muñoz, N. B., & WaiShing, Y. (2017). Using genomic information to improve soybean adaptability to climate change. Journal of Experimental Botany, 68(8), 1823-1834. https://doi.org/10.1093/jxb/erw348. DOI: https://doi.org/10.1093/jxb/erw348
Maranna, S., Nataraj, V., Kumawat, G., Chandra, S., Rajesh, V., Ramteke, R., Patel, R. M., Ratnaparkhe, M. B., Husain, S. M., Gupta, S., Khandekar, N. (2021). Breeding for higher yield, early maturity, wider adaptability and waterlogging tolerance in soybean (Glycine max L.): A case study. Science Report, 11, 22853. https://doi.org/10.1038/s41598-021-02064-x. DOI: https://doi.org/10.1038/s41598-021-02064-x
McCormick, R. F., Truong, S. K., Sreedasyan, A., Kenhins, J., Shu, S., Sims, D., Kennedy, M., Amirebrahimi, M., Weers, B. D., McKinley, B., Mattison, A., Morishige, D. T., Grimwood, J., Schmutz, J., Mullet, J. E. (2018). The Sorghum bicolor reference genome: improved assembly, gene annotations, a transcriptome atlas, and signatures of genome organization. The Plant Journal, 93(2), 338-354. https://doi.org/10.1111/tpj.13781 DOI: https://doi.org/10.1111/tpj.13781
Milioli, A. S., Zdziarski, A. D., Woyann, L. G., Santos, R., Rosa, A. C., Madureira, A., & Benin, G. (2018). Yield stability and relationships among stability parameters in soybean genotypes across years. Chilean Journal of Agricultural Research, 78(2), 299-309. http://dx.doi.org/10.4067/S0718-58392018000200299 DOI: https://doi.org/10.4067/S0718-58392018000200299
Modgil, R., & Goyal, A (2021). Soybean (Glycine max). In: Tanwar, B., Goyal, A. (eds) Oilseeds: Health Attributes and Food Applications. Springer, Singapore. https://doi.org/10.1007/978-981-15-4194-0_17. DOI: https://doi.org/10.1007/978-981-15-4194-0_1
Mohammadi, R., Jafarzadeh, J., Armion, M., Hatamzadeh, H., & Roohi, E. (2023). Clustering stability methods towards selecting best performing and stable durum wheat genotypes. Euphytica, 219(10), 109. https://doi.org/10.1007/s10681-023-03237-7 DOI: https://doi.org/10.1007/s10681-023-03237-7
Mulugeta, B., Tesfaye, K., Geleta, M., Johansson, E., Hailesilassie, T., Hammenhag, C., Hailu, F., & Ortiz, R. (2022). Multivariate analyses of Ethiopian durum wheat revealed stable and high yielding genotypes. PloS One, 17(8), e0273008. https://doi.org/10.1371/journal.pone.0273008 DOI: https://doi.org/10.1371/journal.pone.0273008
Oladosu, Y., Rafii, M. Y., Abdullah, N., Magaji, U., Miah, G., Hussin, G., & Ramli, A. (2017). Genotype × environment interaction and stability analyses of yield and yield components of established and mutant rice genotypes tested in multiple locations in Malaysia. Acta Agriculturae Scandinavica, Section B - Soil & Plant Science, 67(7), 590-606. https://doi.org/10.1080/09064710.2017.1321138 DOI: https://doi.org/10.1080/09064710.2017.1321138
Olivoto, T., Lúcio, A. D. C., Silva, J. A. G., Marchioro, V. S., Souza, V. Q., & Jost, E. (2019). Mean performance and stability in multi-environment trials I: Combining features of AMMI and BLUP techniques. Agronomy Journal, 111(6), 2949-2960. https://doi.org/10.2134/agronj2019.03.0220 DOI: https://doi.org/10.2134/agronj2019.03.0220
Olivoto, T., Lúcio, A. D’C. (2020). Metan: An R package for multi-environment trial analysis. Methods in Ecology and Evolution, 11(6), 783-789. https://doi.org/10.1111/2041-210X.13384 DOI: https://doi.org/10.1111/2041-210X.13384
Piepho, H. -P. (1994). Best linear unbiased prediction (BLUP) for regional yield trials: a comparison to additive main effects and multiplicative interaction (AMMI) analysis. Theoretical and Applied Genetics, 89, 647-654. https://doi.org/10.1007/BF00222462 DOI: https://doi.org/10.1007/BF00222462
Pour-Aboughadareh, A., Khalili, M., Poczai, P., & Olivoto, T. (2022). Stability indices to deciphering the genotype-by-environment interaction (GEI) effect: An applicable review for use in plant breeding programs. Plants, 11(3), 414. https://doi.org/10.3389/fgene.2022.1090994 DOI: https://doi.org/10.3390/plants11030414
Rani, R., Raza, G., Ashfaq, H., Rizwan, M., Shimelis, H., Tung, M. H., & Arif, M. (2023). Analysis of genotype × environment interactions for agronomic traits of soybean (Glycine max [L.] Merr.) using association mapping. Frontiers in Genetics, 13, 1090994. https://doi.org/10.3389/fgene.2022.1090994 DOI: https://doi.org/10.3389/fgene.2022.1090994
Raza, A., Razzaq, A., Mehmood, S. S., Zou, X., Zhang, X., Lv, Y., & Xu, J. (2019). Impact of climate change on crops adaptation and strategies to tackle its outcome: A review. Plants, 8, 34. https://doi.org/10.3390/plants8020034. DOI: https://doi.org/10.3390/plants8020034
Reddy, S. S., Singh, G. M., Kumar, U., Bhati, P., Vishwakarma, M., Navathe, S., Yashavanthakumar, K. J., Mishra, V. K., Sharma, S., & Joshi, A. K. (2024). Spatio-temporal evaluation of drought adaptation in wheat revealed NDVI and MTSI as powerful tools for selecting tolerant genotypes. Field Crops Research, 311, 109367. https://doi.org/10.1016/j.fcr.2024.109367 DOI: https://doi.org/10.1016/j.fcr.2024.109367
Rubiales, D., MORAL, A., & Flores, F. (2022). Agronomic performance of broomrape resistant and susceptible faba bean accession. Agronomy, 12(6), 1421. https://doi.org/10.3390/agronomy12061421 DOI: https://doi.org/10.3390/agronomy12061421
Schoving, C., Champolivier, L., Maury, P., & Debaeke, P. (2022). Combining multi-environmental trials and crop simulation to understand soybean response to early sowings under contrasting water conditions. European Journal of Agronomy, 133, 126439. https://doi.org/10.1016/j.eja.2021.126439 DOI: https://doi.org/10.1016/j.eja.2021.126439
Sharifi, P., Erfani, A., Abbasian, A., & Mohaddesi, A. (2020). Stability of some of rice genotypes based on WAASB and MTSI indices. Iranian Journal of Genetics & Plant Breeding, 9(2).
Shilpashree, N., Devi, S. N., Manjunathagowda, D. C., Muddappa, A., Abdelmohsen, S. A. M., Tamam, N., Elansary, H. O., El-Adedin, T. K. Z., Abdelbacki, A. M. M., & Janhavi, V. (2021). Morphological characterization, variability and diversity among vegetable soybean (Glycine max L.) genotypes. Plants, 10, 671. https://doi.org/10.3390/plants10040671. DOI: https://doi.org/10.3390/plants10040671
Shojaei, S. H., Mostafavi, K., Ghasemi, S. H., Bihamta, M. R., Illés, À., Bojtor, C., Nagy, J., Harsànyi, E., Vad, A., Széles, A., & Mousavi, S. M. N. (2023). Sustainability on Different Canola (Brassica napus L.) Cultivars by GGE biplot graphical technique in multi-environment. Sustainability, 15(11), 8945. https://doi.org/10.3390/su15118945 DOI: https://doi.org/10.3390/su15118945
Singamsetti, A., Shahi, J. P., Zaidi, P. H., Seetharam, K., Vinayam, M. T., Kumar, M., Singla, S., Shikha, K., & Madankar, K. (2021). Genotype × environment interaction and selection of maize (Zea mays L.) hybrids across moisture regimes. Field Crops Research, 270, 108224. https://doi.org/10.1016/j.fcr.2021.108224 DOI: https://doi.org/10.1016/j.fcr.2021.108224
Taleghani, D., Rajabi, A., Saremirad, A., & Fasahat, P. (2023). Stability analysis and selection of sugar beet (Beta vulgaris L.) genotypes using AMMI, BLUP, GGE biplot and MTSI. Scientific Reports, 13(1), 10019. https://doi.org/10.1038/s41598-023-37217-7 DOI: https://doi.org/10.1038/s41598-023-37217-7
Teressa, T., Semahegn, Z., & Bejiga, T. (2021). Multi environments and genetic-environmental interaction (GxE) in plant breeding and its challenges: a review article. International Journal of Research Studies in Agricultural Sciences, 7(4), 11-18. DOI: https://doi.org/10.20431/2454-6224.0704002
Tshikunde, N. M., Mashilo, J., Shimelis, H., & Odindo, A. (2019). Agronomic and physiological traits, and associated quantitative trait loci (QTL) affecting yield response in wheat (Triticum aestivum L.): a review. Frontiers in Plant Science, 10, 1428. https://doi.org/10.3389/fpls.2019.01428 DOI: https://doi.org/10.3389/fpls.2019.01428
Tyagi, S. K., & Tripathi, R. P. (1983). Effect of temperature on soybean germination. Plant and Soil, 74, 273-280. https://doi.org/10.1007/BF02143617 DOI: https://doi.org/10.1007/BF02143617
Vaughan, M. M., Block, A., Christensen, S. A., Allen, L. H., & Schmelz, E. A. (2018). The effects of climate change have linked abiotic stresses to corn phytochemical defenses. Phytochemistery Reviews, 17, 37-49. https://doi.org/10.1007/s11101-017-9508-2 DOI: https://doi.org/10.1007/s11101-017-9508-2
Verma, A., & Singh, G. P. (2021). Superiority index based on AMMI and yield of wheat genotypes evaluated in north western plains zone under restricted irrigated timely sown conditions. 73-82. DOI: https://doi.org/10.15740/HAS/IJAS/17.1/73-82
Vogel, J. T., Liu, W., Olhoft, P., Crafts-Brandner, S., Pennycooke, J. C., & Christiansen, N. (2021). Soybean yield formation physiology–a foundation for precision breeding based improvement. Frontiers in Plant Science, 12, 719706. https://doi.org/10.3389/fpls.2021.719706 DOI: https://doi.org/10.3389/fpls.2021.719706
Wang, R., Wang, H., Huang, S., Zhao, Y., Chen, E., Li, F., Qin, L., Yang, Y., Guan, Y., Liu, B., & Zhang, H. (2023). Assessment of yield performances for grain sorghum varieties by AMMI and GGE biplot analyses. Frontiers in Plant Science, 14, 1261323. https://doi.org/10.3389/fpls.2023.1261323 DOI: https://doi.org/10.3389/fpls.2023.1261323
Wheeler, T., & Braun, J. (2013). Climate change impacts on global food security. Science, 341(6145), 508-513. https://doi.org/10.1126/science.1239402 DOI: https://doi.org/10.1126/science.1239402
Xu, Y., Liu, X., Fu, J., Wang, H., Wang, J., Huang, C., Prasanna, B. M., Olsen, M. S., Wang, G., & Zhang, A. (2020). Enhancing genetic gain through genomic selection: from livestock to plants. Plant Communications, 1(1), 1-21. https://www.cell.com/plant-communications/pdf/S2590-3462(19)30005-7.pdf DOI: https://doi.org/10.1016/j.xplc.2019.100005
Yan, W., Hunt, L. A., Sheng, Q., & Szlavnics, Z. (2000). Cultivar evaluation and mega‐environment investigation based on the GGE biplot. Crop Science, 40(3), 597-605. https://doi.org/10.2135/cropsci2000.403597x DOI: https://doi.org/10.2135/cropsci2000.403597x
Yan, W. (2001). GGEbiplot - A windows application for graphical analysis of multienvironment trial data and other types of two - way data. Agronomy Journal, 93(5), 1111-1118. https://doi.org/10.2134/agronj2001.9351111x DOI: https://doi.org/10.2134/agronj2001.9351111x
Yan, W., & Tinker, N. A. (2005). An integrated biplot analysis system for displaying, interpreting, and exploring genotype x environment interaction. Crop Science, 45(3), 1004-1016. https://doi.org/10.2135/cropsci2004.0076 DOI: https://doi.org/10.2135/cropsci2004.0076
Yan, W., & Tinker, N. A. (2006). Biplot analysis of multi-environment trial data: Principles and applications. Canadian Journal of Plant Science, 86(3), 623-645. https://doi.org/10.4141/P05-169 DOI: https://doi.org/10.4141/P05-169
Yue, H., Gauch, H. G., Wei, J., Xie, J., Chen, S., Peng, H., Bu, J., & Jiang, X. (2022). Genotype by environment interaction analysis for grain yield and yield components of summer maize hybrids across the huanghuaihai region in China. Agriculture, 12(5), 602. https://doi.org/10.3390/agriculture12050602 DOI: https://doi.org/10.3390/agriculture12050602
Zachary, S., Singer, W. M., & Zhang, B. (2020). Soybean production, versability and improvement. In: A legume crops: Prospect, production and uses. IntechOpen, Chapter 3, 182 p.
Zanon, A. J.., Streck, N. A., & Grassini, P. (2016). Climate and management factors influence soybean yield potential in a subtropical environment. Agronomy Journal, 108(4), 1447-1454. https://doi.org/10.2134/agronj2015.0535 DOI: https://doi.org/10.2134/agronj2015.0535
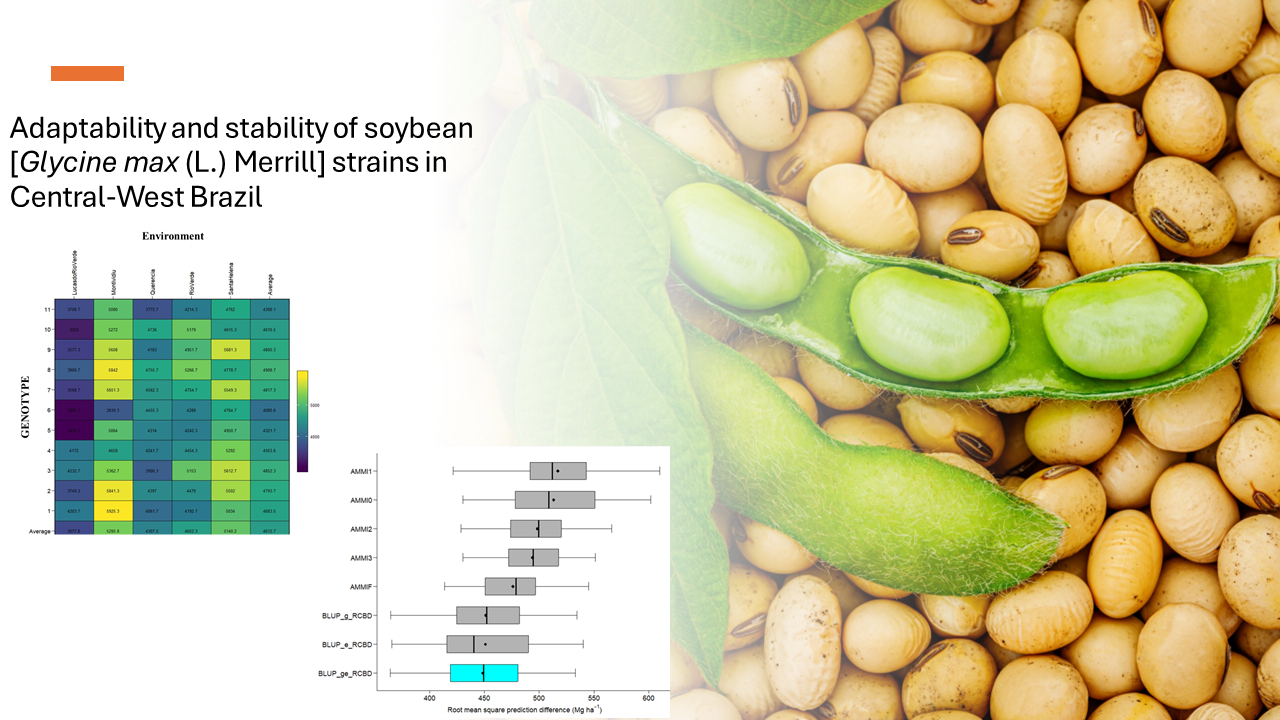
Downloads
Published
How to Cite
Issue
Section
License
Copyright (c) 2024 Wanderson Barbosa da Silva, Antonio Carlos Pereira de Menezes Filho, Mateus Neri Oliveira Reis, Samuel Leandro Soares, Ivandro Bertan, Cláudio Roberto Cardoso de Godoi, Mônica Christina Ferreira, Anaisa Kato Cavalcante, João Carlos Silva Ferreira, Matheus Vinícius Abadia Ventura

This work is licensed under a Creative Commons Attribution 4.0 International License.
Authors who publish with this journal agree to the following terms:
1) Authors retain copyright and grant the journal right of first publication with the work simultaneously licensed under a Creative Commons Attribution License that allows others to share the work with an acknowledgement of the work's authorship and initial publication in this journal.
2) Authors are able to enter into separate, additional contractual arrangements for the non-exclusive distribution of the journal's published version of the work (e.g., post it to an institutional repository or publish it in a book), with an acknowledgement of its initial publication in this journal.
3) Authors are permitted and encouraged to post their work online (e.g., in institutional repositories or on their website) prior to and during the submission process, as it can lead to productive exchanges, as well as earlier and greater citation of published work.